The Rise of Generative AI: A Look at Emerging Trends and Future Implications
Generative AI has experienced a significant rise in recent years, revolutionizing various industries such as art, music, and content creation. The emergence of advanced generative models like GANs (Generative Adversarial Networks) has enabled the generation of highly realistic and creative outputs, pushing the boundaries of what AI can accomplish. Looking ahead, the future implications of generative AI are vast, with potential applications in fields such as personalized medicine, virtual reality, and even the creation of synthetic humans, raising ethical considerations and the need for responsible development and deployment.
Besides, the generative AI market is worth $13.17 million as of 2023. By the end of the year 2025, it is expected to reach a worth of $22.12 billion.
Brief overview of generative AI and its significance in the current tech landscape.
Generative AI refers to a category of artificial intelligence techniques that involve generating new content, such as images, videos, text, or even music, based on patterns and examples from existing data. These models use complex algorithms and deep learning techniques to learn and mimic the patterns and characteristics of the input data and then generate new output that closely resembles the original data.
Generative AI has gained significant prominence in the current tech landscape due to its various applications and potential. Here are some areas where generative AI has demonstrated its significance:
Content Generation
Generative AI models have shown remarkable capabilities in generating realistic and high-quality content. For example, they can generate realistic images of people who do not exist, compose music in various genres, and generate human-like text. This has implications in creative industries, where generative AI can assist artists, writers, and designers in producing new and innovative content.
Data Augmentation
Generative AI techniques can augment existing datasets by generating synthetic data that closely resembles the original data. This helps in increasing the diversity and size of training data, which is particularly useful in domains where collecting large amounts of real-world data is challenging or expensive. Data augmentation improves the performance and generalization ability of machine learning models.
Personalization
Generative AI enables personalized experiences by creating customized content based on individual preferences and characteristics. This is evident in recommender systems that generate personalized product recommendations, content curation algorithms that tailor news and social media feeds to user interests, and virtual assistants that generate natural language responses based on user queries.
Simulation and Training
Generative AI models can be used to simulate and generate realistic environments for training purposes. For example, in robotics, generative AI can simulate different scenarios to train robots in a virtual environment before deploying them in the real world. This reduces costs and risks associated with physical experimentation and enables faster development and iteration of AI systems.
Creative Tools and Design
Generative AI can act as a creative tool for designers and architects. By providing inputs and constraints, designers can use generative AI models to explore a wide range of design possibilities and automatically generate design options that meet specific criteria. This assists in the rapid prototyping and ideation process.
Check out our article on how to develop a product with ChatGPT
Rapid evolution of generative AI and its potential impact on various industries
Generative artificial intelligence (AI) has undergone rapid evolution in recent years, revolutionizing various industries and unlocking new possibilities. With advancements in deep learning and neural networks, generative AI models have become increasingly adept at creating original and realistic content, ranging from text and images to music and videos. This breakthrough technology holds immense potential for industries such as entertainment, marketing, and design, where creative content plays a crucial role. Generative AI enables the automated generation of engaging, personalized content, empowering businesses to deliver unique experiences to their customers at scale.
The impact of generative AI extends beyond content creation, reaching into sectors like healthcare and manufacturing. In healthcare, generative AI models have shown promise in medical image analysis, drug discovery, and personalized medicine. By analyzing vast amounts of data, these models can assist in diagnosing diseases, designing more effective drugs, and tailoring treatments to individual patients. Similarly, in manufacturing, generative AI can optimize processes, predict equipment failures, and even design new products. The ability to generate and simulate countless design variations empowers manufacturers to rapidly prototype and iterate, leading to improved efficiency and innovation.
While the rapid evolution of generative AI brings numerous benefits, it also raises important ethical considerations. There is a growing demand for transparency and accountability in AI systems to address biases and maintain trust. To fully harness the potential of generative AI, it is crucial for industry stakeholders, policymakers, and researchers to collaborate in establishing guidelines that balance innovation with ethical considerations, fostering a future where generative AI benefits society as a whole.
The Evolution of Generative AI
Generative AI has progressed from basic models capable of generating simple images and text to advanced systems that can create highly realistic and diverse outputs across various domains such as art, music, and even human-like conversations. This evolution has been driven by advancements in deep learning architectures, training techniques, and the availability of vast amounts of data, enabling generative AI to push the boundaries of creativity and imagination.
Historical perspective on the development of generative AI
The development of generative AI has witnessed remarkable milestones and breakthroughs over the years, revolutionizing various fields such as computer vision, natural language processing, and art. One of the earliest notable milestones in this domain was the introduction of Markov chain models in the 1950s. These models utilized probabilistic methods to generate sequences of symbols, laying the foundation for subsequent advancements.
In the late 1980s, the introduction of recurrent neural networks (RNNs) brought significant progress to generative AI. RNNs allowed for the modeling of sequential data by maintaining an internal memory, making them capable of generating coherent and context-aware outputs. This development paved the way for applications such as speech recognition and language modeling.
Another key breakthrough came in 2014 with the introduction of Generative Adversarial Networks (GANs). Proposed by Ian Goodfellow, GANs presented a novel approach to generative modeling by involving a generator network and a discriminator network in a competitive setting. The generator network aimed to produce realistic outputs, while the discriminator network learned to distinguish between real and generated samples. The adversarial training between these networks led to significant advancements in generating realistic images, audio, and even text.
In 2019, OpenAI's GPT-2 model marked another milestone in generative AI. GPT-2 demonstrated unprecedented capabilities in natural language generation and understanding. By utilizing a transformer architecture and training on a vast corpus of text data, GPT-2 was able to generate coherent and contextually relevant text, often indistinguishable from human-written content. This breakthrough sparked both excitement and concerns about the potential misuse of AI-generated text.
Current state of generative AI
Generative AI has witnessed remarkable advancements in recent years, pushing the boundaries of what machines can create. One notable breakthrough is the development of deep learning models such as OpenAI's GPT-3, and later on GPT-4, which has the ability to generate human-like text based on given prompts. GPT-3 and GPT-4 have been used in various applications, including creative writing, chatbots, and language translation. Its impressive performance showcases the potential of generative AI in enhancing productivity and transforming industries.
Another significant advancement in generative AI is the emergence of realistic image synthesis models. Deep learning architectures like StyleGAN2 have demonstrated the ability to generate high-resolution images that are indistinguishable from real photographs. This has profound implications for the entertainment industry, as it enables the creation of lifelike computer-generated characters and environments for movies, video games, and virtual reality experiences. Moreover, generative AI has also found applications in fields like fashion, interior design, and advertising, where it can generate photorealistic images of products before they are physically produced.
Power of Adaptability
The power of adaptability in generative AI lies in its ability to learn from and respond to dynamic and evolving environments. By continuously analyzing and adjusting its output based on real-time feedback, generative AI can rapidly adapt its creative outputs to meet changing needs and preferences. This adaptability enables generative AI to stay relevant, innovative, and versatile across various domains, from art and music to language and problem-solving.
Adaptability of generative AI and it's integration into everyday applications
Generative AI has shown remarkable adaptability and is increasingly being integrated into everyday applications, revolutionizing various industries. One area where generative AI excels is in content creation. Whether it's generating realistic images, music, or even entire virtual environments, generative AI models have demonstrated the ability to produce high-quality, original content. For example, in the gaming industry, developers can use generative AI to create realistic landscapes, characters, and animations, saving time and resources in the design process.
Another area where generative AI is making significant strides is in natural language processing and text generation. Language models like GPT-3 have proven their versatility in tasks such as generating coherent and contextually appropriate text, answering questions, and even creating conversational agents. This adaptability has led to the integration of generative AI into customer service chatbots, virtual assistants, and language translation tools, enhancing user experiences and providing more efficient and accurate services.
Generative AI is also being leveraged in the field of healthcare. Medical image synthesis, for instance, allows generative AI models to generate realistic medical images based on limited or incomplete data, aiding in the diagnosis and treatment of various conditions. Additionally, generative AI is being used to simulate drug interactions and optimize drug discovery processes, potentially accelerating the development of new medications and treatments.
Furthermore, generative AI is finding its way into creative industries like fashion and design. Fashion designers can employ generative AI to create unique designs, explore new aesthetics, and even generate virtual fashion models for virtual try-on experiences. In architecture, generative AI is being utilized to generate innovative building designs based on specific criteria, leading to more efficient and sustainable structures.
According to the European AI Alliance, the potential to improve the effectiveness and quality of public digital services by incorporating generative AI into their design is enormous. The once-only principle, user-centered design, accessibility, transparency, security, and adaptability are just a few of the fundamental guidelines that public administrations should follow when utilizing generative AI to develop novel, user-friendly solutions that are advantageous to both individuals and companies.
Generative AI demonstrates remarkable adaptability and is being integrated into a wide range of everyday applications. From content creation and natural language processing to healthcare and creative industries, generative AI is enhancing productivity, efficiency, and creativity. However, as this technology progresses, it is essential to address ethical considerations and ensure responsible and beneficial use of generative AI in our society.
How users utilize AI, including ChatGPT and other AI tools
AI, including ChatGPT and other AI tools, is increasingly designed to meet users where they are, tailoring experiences to their specific needs and preferences. One notable example is the integration of AI assistants in messaging platforms and chat applications. ChatGPT, for instance, can be embedded in popular messaging apps, allowing users to seamlessly interact with the AI model within their preferred communication channels. This integration enables users to access AI-powered features, such as language translation, smart suggestions, or even personalized recommendations, all without leaving the conversation.
Another way AI is meeting users where they are is through voice-activated virtual assistants. These AI tools, like Amazon's Alexa or Google Assistant, have become a ubiquitous presence in homes and mobile devices. By leveraging natural language processing and speech recognition capabilities, these assistants allow users to interact with technology simply by speaking, making it easier for people to access information, perform tasks, and control their smart devices without the need for manual input.
Here you can read more on AI code completion for product development.
Multimodal Generative AI
Using multimodal generative AI, researchers have developed a groundbreaking system that combines text and images to generate highly realistic and contextually coherent descriptions of visual content, bridging the gap between language and vision. This innovative technology opens up new possibilities for immersive storytelling, interactive gaming experiences, and enhanced human-computer interaction.
Concept of multimodal generative AI and its potential for creating diverse outputs
Multimodal generative AI refers to a branch of artificial intelligence that combines multiple modes of data, such as images, text, and audio, to generate diverse and coherent outputs. Traditional AI models usually operate on a single modality, such as text or images, but multimodal generative AI enables the integration of different modalities to produce more comprehensive and nuanced results.
By incorporating various modalities, multimodal generative AI systems can capture richer contextual information and generate outputs that are more diverse and realistic. For example, a multimodal language model can take both text and image inputs and generate detailed and descriptive captions that accurately reflect the content of the images. This allows for a more immersive and engaging user experience.
The potential of multimodal generative AI lies in its ability to leverage the complementary strengths of different modalities. By combining text, images, and audio, these models can generate outputs that transcend the limitations of any single modality. For instance, a multimodal AI system could generate a storybook by taking input from a user's voice commands, analyzing the images on the screen, and dynamically generating text that aligns with the user's narrative choices and the visual context. This opens up exciting possibilities for applications in areas such as content creation, virtual reality, and personalized user experiences.
Moreover, multimodal generative AI also fosters diversity in its outputs. By integrating multiple modalities, the system can generate a wide range of outputs that reflect different perspectives and styles. For example, a multimodal AI model trained on diverse datasets containing various cultural references can produce outputs that cater to different tastes and preferences. This promotes inclusivity and ensures that the generated content resonates with a broader audience.
Overall, multimodal generative AI offers a powerful approach for creating diverse outputs by leveraging multiple modalities. By integrating text, images, and audio, these models can generate outputs that are more comprehensive, realistic, and contextually grounded. The potential applications of multimodal generative AI are vast, ranging from content creation and storytelling to personalized user experiences, and they have the potential to enhance various aspects of our digital interactions and entertainment.
Recent developments in multimodal AI
Multimodal AI, which combines multiple modalities such as text, images, and videos, has seen significant advancements in recent years. Two notable developments in this field are Visual ChatGPT and the rumored features of GPT-4.
Visual ChatGPT: Visual ChatGPT is an extension of the GPT architecture that incorporates visual inputs alongside textual inputs, enabling more immersive and interactive conversational experiences. This model takes both textual prompts and images as inputs and generates responses accordingly. The integration of visual information enhances the AI's ability to understand and generate contextually relevant responses. OpenAI has released an API for Visual ChatGPT, allowing developers to integrate this multimodal AI into their applications.
The introduction of Visual ChatGPT opens up several exciting possibilities across various domains. For example, in customer support applications, users can now provide screenshots or images along with their queries, making it easier for the AI to understand and provide accurate responses. It also enables richer and more interactive virtual assistant experiences, where users can interact with the AI using both text and visual cues.
Here are a few anticipated advancements in GPT-4:
- Improved contextual understanding: GPT-4 is expected to have a better grasp of context, allowing it to generate more coherent and contextually appropriate responses. This improvement would enhance its ability to understand complex prompts and generate nuanced outputs.
- Few-shot and zero-shot learning: GPT-4 might offer enhanced capabilities in learning from limited or zero training examples. This feature would enable the model to perform better with minimal supervision, making it more adaptable to new tasks and domains.
- Enhanced control and customization: GPT-4 may provide improved control over generated outputs, allowing users to specify desired attributes or control the style and tone of the responses. This could enable more fine-grained customization and alignment with user preferences.
- Better multimodal integration: GPT-4 might further advance multimodal AI by improving the integration of different modalities, such as text, images, and audio. This could result in more seamless and coherent multimodal generation, enabling applications with enhanced visual and textual understanding.
AI Image Editing
AI image editing utilizes advanced algorithms and machine learning techniques to enhance, modify, or transform images with remarkable precision and efficiency, revolutionizing the field of digital image manipulation. By leveraging AI's ability to analyze and interpret visual data, it empowers users to achieve stunning visual results and explore creative possibilities previously unimaginable.
Rise of AI image editing and its potential for image creation and modification
AI image editing has witnessed a remarkable rise in recent years and has the potential to revolutionize image creation and modification. With the advent of advanced deep learning algorithms, AI-powered image editing tools have become increasingly sophisticated and capable of producing stunning results. These tools can automate and enhance various aspects of image editing, such as color correction, object removal, background manipulation, and even style transfer. By leveraging large-scale datasets and powerful computational resources, AI image editing algorithms can learn intricate patterns and generate visually pleasing images with remarkable efficiency.
One of the significant advantages of AI image editing is its ability to streamline and expedite the creative process. Tasks that once required painstaking manual effort can now be automated, saving valuable time for artists, designers, and photographers. For example, AI-powered tools can automatically remove unwanted objects from images, saving photographers from the tedious task of manual retouching. Additionally, these tools can assist in generating novel artistic effects or transforming images into different styles, enabling artists to explore new creative possibilities. This speed and efficiency brought about by AI image editing can unlock new avenues for creativity and productivity.
Moreover, AI image editing has the potential to democratize image creation and modification by making sophisticated editing techniques accessible to a wider audience. Previously, complex editing tasks were reserved for professionals with specialized skills and expensive software. However, with the rise of AI image editing tools, individuals with limited technical knowledge can achieve professional-grade results. User-friendly interfaces and intuitive controls allow novices to experiment with editing techniques and achieve desired outcomes easily. This accessibility empowers a broader range of users, including hobbyists, social media enthusiasts, and small business owners, to create visually compelling images without the need for extensive training or expensive software licenses.
Recent advancements in AI image editing
In recent years, there have been significant advancements in AI-powered image editing, revolutionizing the way we manipulate and enhance visual content. One notable development is ControlNet, a deep learning model that allows users to easily edit the content of images by manipulating textual descriptions. ControlNet leverages a technique known as "conditional GANs" (Generative Adversarial Networks) to generate realistic and accurate images based on textual instructions. For example, users can specify a description like "turn the daytime scene into a nighttime scene with a full moon," and ControlNet will intelligently transform the image accordingly. This technology opens up exciting possibilities for creative professionals and enthusiasts, enabling them to effortlessly bring their visual ideas to life.
Another significant advancement in AI image editing is Playground AI, a web-based platform that combines the power of AI and deep learning with an intuitive user interface. Playground AI allows users to edit images with various AI models, including style transfer, image super-resolution, and object removal. It provides a user-friendly environment where users can experiment and apply AI techniques to their images without the need for extensive technical expertise. Playground AI's real-time rendering capabilities make it possible to see instant visual feedback, empowering users to explore different editing possibilities quickly and efficiently.
Furthermore, recent advancements in AI image editing have also introduced impressive capabilities in face editing and manipulation. AI models like FaceApp and DeepArt have gained popularity for their ability to transform facial features, age progression/regression, and apply artistic styles to portraits. These models employ deep neural networks to analyze and understand facial structures, allowing users to generate highly realistic and visually appealing modifications to their photos. With the increasing accessibility of such AI-powered tools, users can now effortlessly experiment with various visual effects and transformations, enhancing their creativity and enabling new avenues of self-expression in the digital realm.
Fine-Tuned Customized Models
Fine-tuned customized models in generative AI refer to models that have been specifically trained on a particular dataset or task to improve their performance in generating creative content. These models undergo additional training and fine-tuning to adapt to specific requirements, resulting in more tailored and context-aware outputs. By incorporating domain-specific knowledge and optimizing for specific objectives, these models can generate more accurate and relevant responses in various creative applications.
Trends towards customized models in Generative AI
As the field of AI continues to evolve, businesses and researchers have recognized the value of tailoring models to address specific requirements and challenges. Customized models offer several advantages over general-purpose models by providing enhanced performance, increased efficiency, and improved accuracy.
One key benefit of customized models is their ability to better understand and generate content that aligns with the specific needs of a particular industry or domain. For example, in the field of medicine, customized models can be trained on large datasets of medical records and research papers to generate highly accurate and relevant summaries or diagnoses. Similarly, in the creative industries, customized generative models can be trained on specific artistic styles or genres to produce unique and personalized artworks, music, or storytelling.
Customized models also allow for more efficient and resource-friendly computation. By focusing on specific use cases, the models can be designed to optimize for the particular tasks they are meant to perform. This enables faster training and inference times, reducing the computational requirements and costs associated with running AI models. Furthermore, customized models can be fine-tuned on smaller datasets, which is especially useful when data availability is limited or when dealing with sensitive or proprietary information.
Despite the advantages, there are challenges associated with customized models. Developing a tailored model requires domain expertise and a deep understanding of the specific problem at hand. Data collection and curation may be more complex, as it is crucial to gather representative and high-quality data for training. Additionally, the customization process needs to strike a balance between overfitting to the specific use case and maintaining generalizability to handle diverse scenarios.
Domain-specific models like BioGPT and Galactica, and platforms like Mage.space or Leonardo.ai
Domain-specific models have gained significant attention in recent years due to their ability to cater to specific fields and deliver specialized results. One such example is BioGPT, a domain-specific model developed for the field of biology and bioinformatics. BioGPT is trained on a vast corpus of biological literature and databases, enabling it to generate high-quality text and assist researchers in tasks such as literature review, drug discovery, and protein analysis. By understanding the specific nuances and terminologies of the biological domain, BioGPT proves to be a valuable tool for scientists in the field.
Galactica, on the other hand, is a domain-specific model tailored for astronomy and astrophysics. It is trained on a wide range of astronomical data, including observations, simulations, and theoretical models. Galactica can provide insights into various celestial phenomena, help analyze astronomical images, and even predict the behavior of cosmic objects. This specialized model assists astronomers in their research and enhances our understanding of the universe.
In addition to domain-specific models, platforms like Mage.space and Leonardo.ai have emerged to support and facilitate the use of these models. Mage.space is a collaborative platform designed to accelerate the development of AI models in various domains. It provides a user-friendly interface and infrastructure for training, fine-tuning, and deploying models. Researchers and developers can leverage Mage.space to collaborate, share knowledge, and collectively advance domain-specific AI applications.
Similarly, Leonardo.ai is another platform that focuses on enabling the creation and deployment of AI models. It offers a comprehensive suite of tools and services to build custom models, including domain-specific models. Leonardo.ai provides pre-trained models, transfer learning capabilities, and extensive customization options, making it easier for developers and researchers to create AI solutions for specific industries or use cases.
These domain-specific models and platforms demonstrate the growing trend of tailoring AI technologies to address the unique requirements of specific fields. By catering to the nuances and complexities of specialized domains, these models and platforms empower researchers, professionals, and enthusiasts to leverage the power of AI in their respective areas of expertise.
Rise of Conversational AI
The rise of Conversational AI has revolutionized the way we interact with technology, enabling natural language conversations and personalized user experiences across various applications and industries. Its continuous advancement and integration into various platforms are reshaping the future of human-computer interactions.
Increasing prevalence of conversational AI and its integration into various platforms and applications
Conversational AI, powered by technologies such as natural language processing (NLP) and machine learning, has experienced a significant rise in prevalence over the past few years. This growth can be attributed to several factors. First and foremost, advancements in AI research and computing power have significantly improved the performance and capabilities of conversational AI systems. This has led to more accurate and contextually aware interactions, making it increasingly difficult for users to distinguish between human and AI responses. As a result, businesses and organizations across various industries have recognized the potential of conversational AI in enhancing customer experiences, automating tasks, and streamlining operations.
Another key driver behind the increasing prevalence of conversational AI is its seamless integration into various platforms and applications. Technology giants like Google, Amazon, Microsoft, and Facebook have invested heavily in developing AI-driven chatbots and virtual assistants. These conversational interfaces can now be found in messaging apps, websites, social media platforms, and even smart home devices. By embedding conversational AI into these platforms, businesses can engage with customers on their preferred channels and provide real-time support, leading to improved user satisfaction and brand loyalty.
Furthermore, the rise of open-source AI frameworks and APIs has made it easier for developers and businesses to implement conversational AI in their own applications. This accessibility has democratized the technology, allowing startups and small enterprises to leverage conversational AI without significant upfront costs. As a result, we have witnessed a proliferation of chatbots in various domains, from customer support and e-commerce to healthcare and finance.
The increasing prevalence of conversational AI can be attributed to advancements in AI research, improved computing power, and the seamless integration of these technologies into various platforms and applications. As AI technology continues to evolve, we can expect conversational AI to become even more sophisticated and pervasive, transforming the way we interact with machines and enabling more efficient and personalized experiences across different domains. However, it is essential to address privacy and ethical concerns associated with AI to ensure that these technologies are used responsibly and for the benefit of society as a whole.
Potential of multimodal chatbots and the future of conversational AI
The potential of multimodal chatbots is incredibly promising and represents the future of conversational AI. By integrating multiple modes of communication, such as text, voice, images, videos, and even gestures, multimodal chatbots can deliver a more engaging and personalized user experience. This allows them to better understand user intent, context, and emotions, leading to more natural and effective interactions. For example, users can seamlessly switch between voice commands and text inputs, making conversations more fluid and intuitive. Moreover, multimodal chatbots can leverage visual and auditory information to provide richer responses, enabling them to assist with tasks that were previously challenging for traditional chatbots. With the growing availability of smart devices and technologies, multimodal chatbots have the potential to become ubiquitous in various domains, including customer support, healthcare, education, and entertainment.
Looking ahead, the future of conversational AI is expected to be characterized by ever-improving capabilities and human-like interactions. As natural language processing (NLP) and computer vision technologies advance, chatbots will become even better at understanding and generating human language and interpreting visual cues. This will lead to chatbots that can engage in more meaningful and complex conversations, provide context-aware responses, and adapt their behavior based on user preferences. Additionally, the integration of machine learning and deep learning techniques will enable chatbots to continuously learn from user interactions, enhancing their ability to personalize responses and cater to individual needs. Furthermore, as chatbots become more sophisticated, they are likely to collaborate with humans in more collaborative and productive ways, transforming the way we work, learn, and communicate. Overall, the future of conversational AI and multimodal chatbots holds immense potential to revolutionize human-computer interactions and redefine how we interact with technology in our daily lives.
Future of Generative AI
The future of generative AI holds tremendous potential to revolutionize various industries, from art and entertainment to healthcare and manufacturing. As research continues to advance, we can expect even more sophisticated and versatile AI models capable of generating realistic and creative content, pushing the boundaries of what's possible in the realm of artificial intelligence.
Potential future trends in generative AI: risks and ethical considerations
In the future, generative AI is expected to witness significant advancements and trends. One potential trend is the development of even more powerful and sophisticated models capable of generating highly realistic and contextually coherent content across various domains, such as text, images, and videos. As the technology improves, we may see AI-generated content becoming indistinguishable from human-created content, leading to new challenges in verifying the authenticity of information and media. Additionally, generative AI is likely to play a crucial role in personalized content creation, tailoring products, services, and experiences to individual preferences, thereby enhancing user engagement and satisfaction.
However, along with these promising advancements, there are substantial risks and ethical considerations associated with generative AI. One significant concern is the potential misuse of the technology for malicious purposes, such as generating deep fake content for spreading misinformation, propaganda, or fraudulent activities. There will be a need for robust and effective methods to detect and counteract such misuse. Furthermore, as AI systems become more capable, there could be heightened privacy concerns as these systems may have access to vast amounts of user data. Addressing issues of data privacy, and algorithmic bias, and ensuring transparency in AI decision-making will be crucial to ensure that generative AI benefits society without amplifying existing inequalities or undermining human rights. It will be essential for policymakers, researchers, and developers to work collaboratively in establishing ethical guidelines and regulations to steer generative AI's future development responsibly.
Role of generative AI in drug discovery, material science, chip design, synthetic data, and generative design of parts
Generative AI plays a crucial role in various fields, including drug discovery, material science, chip design, synthetic data generation, and the generative design of parts. In drug discovery, generative AI models such as Generative Adversarial Networks (GANs) and Variational Autoencoders (VAEs) can assist in generating novel molecular structures and predicting their properties. By generating and analyzing vast libraries of potential compounds, researchers can accelerate the process of identifying promising drug candidates, saving both time and resources in the early stages of drug development.
In material science, generative AI techniques enable the design of new materials with specific properties. By utilizing machine learning algorithms and simulations, researchers can explore the vast space of potential materials and identify compositions that exhibit desired characteristics, such as high strength, conductivity, or thermal stability. This approach opens up possibilities for creating advanced materials for various applications, ranging from electronics to aerospace.
In chip design, generative AI can optimize the layout and architecture of complex integrated circuits. By leveraging neural networks and reinforcement learning, designers can automate the design process, leading to improved performance, reduced power consumption, and faster time-to-market for semiconductor products. Generative AI allows for exploration of design alternatives that would be impractical to explore manually, leading to more efficient and powerful microchips.
Moreover, generative AI plays a significant role in generating synthetic data, which is essential in cases where real data is scarce or sensitive. These synthetic datasets can be used to train machine learning models without compromising privacy or security. Generative models can create realistic data that retains statistical properties of the original dataset while eliminating any personally identifiable information. This synthetic data enables researchers and developers to create more robust and generalized AI models.
Lastly, generative design of parts involves using AI-driven algorithms to optimize the design of components for various industries, such as automotive, aerospace, and manufacturing. By defining design objectives and constraints, generative AI can explore a vast array of potential designs and produce optimal solutions that meet specific performance criteria. This approach leads to highly efficient and lightweight parts, reducing material waste and improving overall product performance.
In conclusion, generative AI revolutionizes various aspects of scientific and engineering disciplines, from drug discovery and material science to chip design, synthetic data generation, and generative design of parts. By harnessing the power of AI-driven creativity and optimization, these fields can experience faster advancements, increased efficiency, and breakthrough innovations that have a profound impact on society.
Conclusion
In conclusion, Generative AI stands at the forefront of cutting-edge technology, revolutionizing various industries and reshaping the way we interact with machines. The rapid advancements in this field have given rise to remarkable applications, such as text generation, image synthesis, music composition, and even virtual world creation. With the ability to produce highly realistic and creative outputs, Generative AI has unlocked immense potential for artists, designers, and developers, offering them a novel toolset to augment their creativity and productivity.
However, as with any powerful technology, Generative AI also brings forth ethical and societal considerations. The risk of deep fakes and misinformation looms large, necessitating responsible use and regulation to ensure the technology's positive impact outweighs its potential pitfalls. Additionally, issues of bias and fairness in the data used to train these models must be carefully addressed to prevent perpetuating harmful stereotypes and inequalities. Despite these challenges, the promise of Generative AI remains undeniable, and with ongoing research, collaboration, and ethical stewardship, this technology will continue to unlock new horizons of human-machine interaction and foster a more innovative and creative world.
And here are some experts’ opinions on AI:
Sissie Hsiao (from Google): It's really here to help you brainstorm ideas, to generate content, like a speech, a blog post, or an email.
Sundar Pichai, CEO of Alphabet Inc. (Google's parent company): "AI is one of the most important things humanity is working on. It is more profound than, I dunno, electricity or fire."
Andrew Ng, Co-founder of Coursera and former Chief Scientist at Baidu: "AI is the new electricity. Just as electricity transformed almost everything 100 years ago, today I actually have a hard time thinking of an industry that I don't think AI will transform in the next several years."
So, staying informed about all the future advancements of generative AI is important for a better understanding of the tech environment and, thus, better decision-making.
you may also want to read
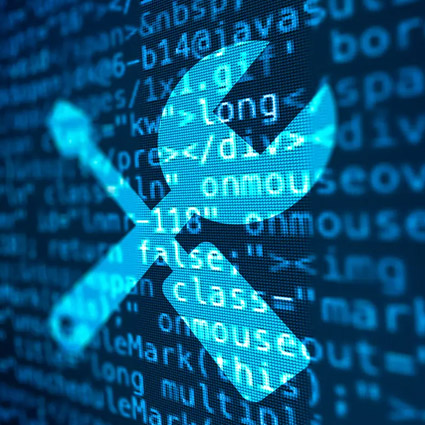
Hire Laravel Framework Developer: Tips and Offers
Do you dream of a dynamic website, a secure eCommerce store, or a new game but lack the resources for...
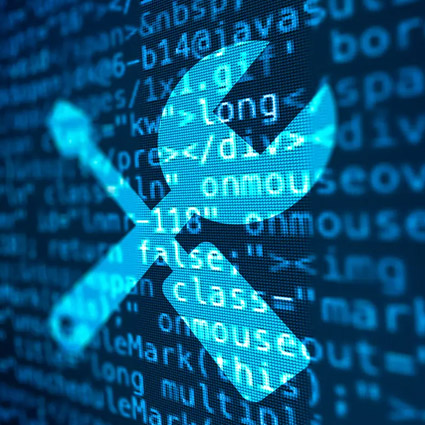
AI in HR: Transforming Human Resources
AI in HR is promised to change the recruitment operational activities dramatically. Despite the changes expected to be grasped by...
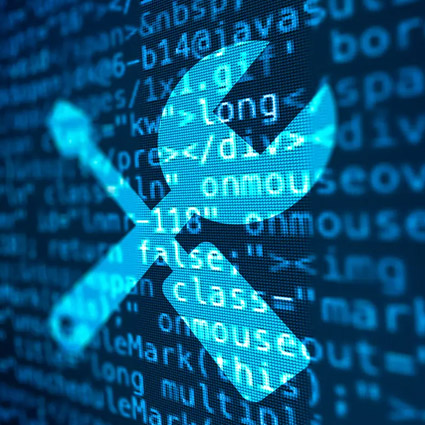
Python Telematic Solutions: Unfolding Capabilities for Fleet Management
The success of transportation and logistics companies is defined by how efficiently their fleet operates. This includes vehicle tracking, route...