Brief explanation of predictive maintenance and IoT
Predictive maintenance is a type of maintenance strategy that uses data analytics techniques to predict when a machine or equipment is likely to fail. This approach is used to reduce unplanned downtime and improve maintenance efficiency by allowing maintenance teams to perform maintenance activities proactively, based on data-driven insights.
Internet of Things (IoT) refers to the network of interconnected devices that are able to collect and exchange data with each other. IoT devices can include sensors, wearables, and other smart devices that are embedded with technology that allows them to connect to the internet and transmit data. In the context of predictive maintenance, IoT devices can be used to collect real-time data from machines and equipment, which can then be used to predict when maintenance is required. By combining predictive maintenance with IoT, organizations can improve their maintenance efficiency and reduce the risk of downtime. Now when we’ve talked about predictive maintenance meaning and IoT, let’s look further at the importance of IoT preventative maintenance.
The importance of predictive maintenance in modern industries
Predictive maintenance is a proactive approach to maintenance that involves using data analysis techniques to predict when equipment is likely to fail and scheduling maintenance activities accordingly. This approach can help modern industries save time and money by reducing unplanned downtime, improving equipment reliability, and extending the lifespan of assets.
Here are some key reasons why predictive maintenance is important in modern industries:
Reduced downtime
By predicting when equipment is likely to fail, organizations can schedule maintenance activities during planned downtime periods, minimizing the impact of equipment failure on production.
Improved equipment reliability
Predictive maintenance can help organizations identify and address issues before they become major problems, preventing equipment breakdowns and extending the lifespan of assets.
Increased safety
Regular maintenance can help ensure that equipment is functioning safely, reducing the risk of accidents and injuries.
Cost savings
Predictive maintenance can help organizations save money by reducing the need for emergency repairs, lowering maintenance costs, and extending the lifespan of assets.
Enhanced efficiency
By optimizing maintenance schedules, organizations can reduce the amount of time and resources needed for maintenance activities, allowing them to focus on other areas of the business.
Understanding IoT and Predictive Maintenance
Predictive maintenance is an approach that aims to optimize maintenance activities by using data analysis techniques to predict when equipment or machinery is likely to fail. Instead of relying on fixed maintenance schedules or waiting for a breakdown, predictive maintenance leverages data from sensors, equipment logs, and other sources to identify patterns, anomalies, and early signs of failure.
By monitoring the condition of equipment in real-time and analyzing historical data, predictive maintenance algorithms can detect potential issues before they cause major problems. This allows maintenance teams to schedule repairs or replacements proactively, reducing downtime, optimizing maintenance costs, and increasing overall operational efficiency.
IoT plays a crucial role in enabling predictive maintenance. IoT devices embedded in machinery and equipment collect real-time data on factors like temperature, vibration, pressure, and usage. This data is then analyzed using machine learning algorithms and predictive models to identify patterns that indicate impending failures or anomalies.
Definition and key features of IoT
The key features of IoT include:
Connectivity
IoT devices are equipped with various communication technologies such as Wi-Fi, Bluetooth, cellular networks, or even specialized low-power protocols to enable seamless data transmission and interaction with other devices or systems.
Sensing and Actuation
IoT devices incorporate sensors and actuators to monitor and collect data from their surrounding environment. Sensors can include temperature sensors, motion sensors, light sensors, and more. Actuators enable the devices to interact with their environment by performing actions based on received data.
Data Analytics
IoT generates a massive amount of data, and analytics play a crucial role in extracting valuable insights from this data. Advanced analytics techniques, including machine learning and artificial intelligence, are applied to analyze the collected data and derive meaningful information.
Automation and Control
IoT devices often aim to automate processes and control systems remotely. By leveraging connectivity and data analytics, they enable remote monitoring, management, and control of various devices and systems, offering enhanced convenience and efficiency.
Scalability and Flexibility
IoT systems can be scaled up or down easily to accommodate a growing number of devices and users. They are designed to be flexible, allowing for the addition of new devices or the modification of existing ones without disrupting the overall system.
Security and Privacy
Given the sensitive nature of the data collected and transmitted by IoT devices, ensuring security and privacy is critical. IoT systems need robust measures to protect against cyber threats, data breaches, and unauthorized access, both at the device level and during data transmission.
These key features collectively enable IoT to create a network of smart, interconnected devices that can improve various aspects of our lives, such as home automation, industrial processes, healthcare monitoring, environmental monitoring, transportation systems, and more.
At A-Team Global, we offer custom IoT solutions that can significantly improve business operational efficiency.
Explanation of predictive maintenance
Predictive maintenance is a proactive maintenance strategy that uses data analysis techniques and advanced technologies to predict when equipment or machinery is likely to fail and requires maintenance. It aims to prevent unexpected breakdowns, reduce downtime, and optimize maintenance activities.
Traditional maintenance approaches typically involve scheduled maintenance at fixed intervals, regardless of the actual condition of the equipment. This can result in unnecessary maintenance or, conversely, equipment failures if the scheduled maintenance is insufficient. Predictive maintenance, on the other hand, relies on real-time monitoring, data collection, and analysis to determine the optimal time for maintenance.
The process of predictive maintenance involves several key steps:
Data collection
Sensors, meters, and other monitoring devices are used to collect various types of data from the equipment. This can include temperature, pressure, vibration, noise, fluid levels, and other relevant parameters. The data is typically collected at regular intervals or continuously.
Data analysis
The collected data is analyzed using advanced analytics techniques, such as machine learning algorithms. Patterns, trends, and anomalies are identified to detect any deviations from normal operating conditions. The analysis aims to find correlations between the collected data and equipment failures or performance degradation.
Fault detection and diagnosis
By comparing the real-time data with historical data and established thresholds, predictive maintenance systems can detect the early signs of equipment faults or failures. This allows for timely intervention before the situation worsens.
Maintenance planning
Once a potential issue is identified, maintenance planners can schedule the appropriate maintenance activities. This can involve ordering replacement parts, allocating resources, and coordinating maintenance personnel. Predictive maintenance enables maintenance to be performed only when necessary, minimizing the disruption to operations and reducing costs.
Proactive maintenance
With the knowledge gained from predictive maintenance, proactive measures can be taken to address potential issues. This can include adjusting operating parameters, implementing temporary workarounds, or applying preventive measures to mitigate the risk of failure.
The benefits of predictive maintenance include increased equipment uptime, optimized maintenance schedules, reduced maintenance costs, improved safety, and enhanced overall operational efficiency. By identifying and addressing maintenance needs in a timely manner, organizations can avoid unplanned downtime, extend equipment lifecycles, and optimize their maintenance resources.
The synergy between IoT and predictive maintenance
The synergy between the Internet of Things (IoT) and predictive maintenance is significant and has the potential to revolutionize maintenance practices in various industries. IoT refers to the network of interconnected devices and sensors that can collect and exchange data over the internet. Predictive maintenance, on the other hand, involves using data and analytics to predict equipment failures or maintenance needs before they occur.
So with IoT preventative maintenance, organizations can achieve several benefits:
Real-time monitoring
IoT sensors can continuously monitor equipment and collect data on various parameters such as temperature, vibration, pressure, and more. This real-time monitoring allows for early detection of anomalies or deviations from normal operating conditions.
Data-driven insights
The vast amount of data collected by IoT devices can be processed and analyzed using advanced analytics techniques. By applying machine learning algorithms to this data, patterns, trends, and potential failure signatures can be identified. This enables maintenance teams to make data-driven decisions and prioritize maintenance activities effectively.
Reduced downtime and costs
Predictive maintenance allows for proactive and preventive maintenance actions. By identifying and addressing potential issues before they lead to failures, organizations can avoid unplanned downtime and costly repairs. This approach helps optimize maintenance schedules and reduce overall maintenance costs.
Remote monitoring and diagnostics
IoT facilitates remote monitoring of equipment, enabling maintenance teams to monitor and diagnose issues from a central location. This capability is particularly valuable for geographically dispersed assets or inaccessible locations, as it reduces the need for physical inspections and improves the speed of response.
Enhanced safety and risk mitigation
Predictive maintenance helps identify potential safety risks or hazards associated with equipment failures. By addressing these issues proactively, organizations can improve workplace safety and reduce the likelihood of accidents or incidents.
You can also check out the article on IoT-based GPS tracking software - https://a-team.global/blog/iot-based-gps-tracking-software/
Advantages of IoT-Enabled Predictive Maintenance
Now let’s look at some advantages of IoT-enabled predictive maintenance.
Increased equipment lifespan
One of the significant advantages of IoT-enabled predictive maintenance is the increased lifespan of equipment. By utilizing IoT sensors, data analytics, and machine learning algorithms, organizations can monitor the health and performance of their equipment in real-time. This proactive approach allows them to identify potential issues and perform necessary maintenance actions before major failures occur. Here's how it contributes to increased equipment lifespan:
Early fault detection
IoT sensors continuously collect data from the equipment, including temperature, vibration, pressure, and other relevant parameters. By analyzing this data in real-time, anomalies and patterns indicative of potential faults or malfunctions can be detected early.
Condition-based maintenance
Instead of following a fixed maintenance schedule or waiting for breakdowns to occur, predictive maintenance relies on the actual condition of the equipment. By monitoring critical parameters and performance metrics, organizations can schedule maintenance activities based on the equipment's real-time health status.
Improved maintenance accuracy
IoT-enabled predictive maintenance utilizes data-driven insights and predictive models to optimize maintenance activities. Maintenance technicians receive accurate information about the condition and potential issues of the equipment, allowing them to perform targeted repairs or replacements.
Enhanced equipment performance
By continuously monitoring equipment performance, organizations can identify opportunities for optimization. IoT sensors can detect deviations from optimal performance, enabling organizations to make necessary adjustments and fine-tune equipment settings. This proactive approach not only increases the equipment's lifespan but also enhances its overall performance, leading to improved productivity and efficiency.
Reduced downtime and maintenance costs
Here's how IoT-enabled predictive maintenance can help reduce downtime and maintenance costs.:
Early fault detection
IoT sensors and devices deployed in machinery and equipment can continuously monitor their operational parameters, such as temperature, vibration, pressure, and performance metrics. By collecting real-time data, anomalies or deviations from normal operating conditions can be detected early on.
Predictive analytics
The collected data from IoT devices is processed and analyzed using advanced analytics techniques, such as machine learning algorithms. By analyzing historical and real-time data patterns, predictive models can be built to forecast potential equipment failures. This allows maintenance teams to schedule maintenance activities based on predicted failure probabilities and prioritize resources accordingly.
Condition-based maintenance
Instead of following a fixed schedule for maintenance activities, IoT-enabled predictive maintenance allows for condition-based maintenance. By monitoring the actual health and performance of equipment in real-time, maintenance can be performed when necessary, based on the condition and usage of the asset. This approach minimizes unnecessary maintenance and optimizes the utilization of maintenance resources.
Optimized maintenance planning
IoT-enabled predictive maintenance provides actionable insights into the health and performance of assets. Maintenance teams can leverage this information to optimize maintenance planning and scheduling. This leads to more efficient maintenance operations and minimizes the impact on regular production activities.
Enhanced operational efficiency
Enhanced operational efficiency is a significant advantage of IoT-enabled predictive maintenance. By leveraging the power of the Internet of Things (IoT) and predictive analytics, organizations can optimize their maintenance practices, leading to improved efficiency across various operational processes.
By implementing IoT-enabled predictive maintenance, organizations can achieve several operational efficiency benefits. First, they can significantly reduce unplanned downtime by addressing issues before they escalate into major failures. This leads to improved productivity and uninterrupted operations, resulting in higher overall equipment effectiveness.
Second, maintenance activities can be streamlined and optimized. Instead of conducting routine inspections and maintenance on all assets, organizations can focus their efforts on those that require attention based on predictive analytics. This targeted approach optimizes the allocation of maintenance resources, reduces costs associated with unnecessary maintenance, and extends the lifespan of critical assets.
Third, IoT-enabled predictive maintenance enables organizations to optimize their inventory management. With accurate predictions of failure probabilities and maintenance needs, spare parts and supplies can be stocked strategically. This prevents overstocking or stockouts, reduces inventory carrying costs, and ensures timely availability of necessary components when maintenance is required.
Improved safety and risk management
Improved safety and risk management is a significant advantage of IoT-enabled predictive maintenance. By harnessing the power of the Internet of Things (IoT) and advanced analytics, organizations can proactively identify and address potential equipment failures or safety hazards before they escalate into major incidents.
With IoT-enabled predictive maintenance, sensors embedded in machines continuously monitor their performance and collect real-time data. This data is then analyzed using sophisticated algorithms to detect patterns, anomalies, and potential failure indicators. By identifying early warning signs, maintenance teams can take timely action to address issues, reducing the risk of accidents, breakdowns, and other safety hazards.
This proactive approach to maintenance enables organizations to move away from reactive and time-based maintenance practices, where equipment is repaired or replaced only after a failure occurs. By predicting and preventing failures, businesses can significantly enhance workplace safety by minimizing the occurrence of sudden breakdowns or malfunctions that could pose risks to employees, customers, or the environment.
Furthermore, IoT-enabled predictive maintenance enables organizations to optimize their maintenance schedules and resource allocation. Instead of conducting routine maintenance activities on fixed schedules, maintenance can be performed when it is truly needed. This not only increases operational efficiency but also reduces the chances of human error or oversight, which can contribute to safety incidents.
Key Components of IoT-Based Predictive Maintenance Systems
Let’s look at the major components of IoT-based predictive maintenance systems
IoT sensors and devices
IoT sensors and devices play a crucial role as key components in IoT-based predictive maintenance systems. Predictive maintenance refers to the practice of using data and analytics to predict when and how equipment or machinery might fail. By leveraging IoT sensors and devices, organizations can monitor the health and performance of their assets in real-time, enabling them to optimize maintenance activities, reduce downtime, and improve operational efficiency.
IoT sensors are small, intelligent devices that collect and transmit data from the physical world to the digital realm. They are capable of measuring various parameters such as temperature, pressure, vibration, humidity, and more. These sensors can be attached to different types of equipment, ranging from industrial machinery to vehicles, appliances, and infrastructure components.
In an IoT-based predictive maintenance system, these sensors are strategically placed on critical components or areas of the equipment to capture relevant data points. The sensors can be integrated into the existing infrastructure or added as retrofitted components. They are designed to be power-efficient, with long battery life or energy harvesting capabilities, ensuring continuous data collection without disrupting the equipment's operation.
Besides sensors, IoT devices such as gateways or edge devices are also essential components of predictive maintenance systems. These devices act as intermediaries between the sensors and the central data processing system. They aggregate the data from multiple sensors, perform basic analytics or preprocessing tasks locally, and transmit the relevant information to the cloud or a centralized server.
IoT devices often incorporate edge computing capabilities, which allow for real-time analysis and decision-making at the edge of the network. This helps reduce latency, bandwidth usage, and dependency on cloud connectivity. Edge computing enables immediate response to critical events or triggers, making it ideal for time-sensitive predictive maintenance scenarios.
Connectivity and communication protocols
Connectivity and communication protocols play a crucial role in IoT-based predictive maintenance systems. These systems leverage the power of the Internet of Things (IoT) to enable real-time monitoring, analysis, and proactive maintenance of various assets and equipment. By utilizing a network of interconnected sensors and devices, organizations can optimize maintenance practices, minimize downtime, and improve operational efficiency.
One of the fundamental requirements of IoT-based predictive maintenance systems is reliable and seamless connectivity. These systems involve the deployment of numerous sensors and devices across a wide area, including factories, industrial plants, or even smart cities. These devices generate a vast amount of data related to the health, performance, and condition of the assets they monitor. To make effective use of this data, it must be transmitted in a timely and secure manner.
Various connectivity options are available for IoT-based predictive maintenance systems, depending on the specific use case and environment. Some commonly used connectivity technologies include: Wireless Connectivity, Cellular Connectivity, LPWAN (Low Power Wide Area Network).
Data storage and processing
Data storage and processing are key components of IoT-based predictive maintenance systems that rely on IoT sensors and devices.
In IoT-based predictive maintenance systems, data storage plays a crucial role in ensuring the availability and accessibility of sensor-generated data. As IoT devices continuously generate a massive volume of data, it is important to have robust and scalable storage solutions capable of handling the influx of information. Cloud-based storage platforms are often utilized, providing flexible and virtually limitless storage capacity. These platforms enable organizations to store and manage their sensor data efficiently, without the need for significant on-premises infrastructure investments.
Furthermore, data processing is essential for extracting valuable insights from the collected data. The raw data obtained from IoT sensors often needs to be transformed, aggregated, and analyzed to derive meaningful information. Data processing techniques, such as data cleansing, filtering, and feature extraction, are applied to ensure data quality and relevance
To facilitate data storage and processing in IoT-based predictive maintenance systems, edge computing is often utilized. Edge computing involves performing data processing and analysis closer to the data source, at the network edge, rather than sending all the data to a centralized cloud infrastructure. This approach reduces latency, optimizes bandwidth usage, and enables real-time or near-real-time decision-making. By deploying edge computing capabilities, organizations can process and store critical data locally, while still leveraging the scalability and computational power of cloud platforms when needed.
Predictive analytics and machine learning algorithms
Two key components that enable the effectiveness of IoT-based predictive maintenance systems are predictive analytics and machine learning algorithms.
In the context of IoT-based predictive maintenance systems, predictive analytics plays a crucial role in processing the vast amount of sensor data collected from IoT devices. It involves analyzing historical data, detecting patterns, and identifying potential anomalies or abnormalities that could indicate equipment failure or maintenance needs.
Machine learning algorithms are an integral part of predictive analytics in IoT-based predictive maintenance systems. These algorithms can be trained using historical data to recognize patterns, correlations, and anomalies that indicate the likelihood of future failures or maintenance requirements. As new data is continuously collected from IoT sensors and devices, machine learning algorithms adapt and improve their predictive capabilities over time, allowing them to make more accurate predictions.
Visualization and reporting tools
IoT-based predictive maintenance systems rely on the continuous monitoring of equipment and machinery through the use of IoT sensors and devices. These systems generate vast amounts of data, providing valuable insights into the performance and health of various assets. However, to make this data actionable and facilitate informed decision-making, visualization and reporting tools play a crucial role.
These predictive maintenance technologies help transform raw data into meaningful visual representations. They allow users to analyze and interpret complex data sets by presenting them in intuitive and interactive formats such as charts, graphs, dashboards, and heat maps. By visualizing data, operators and maintenance personnel can quickly identify patterns, trends, anomalies, and correlations that might indicate potential equipment failures or maintenance requirements.
Reporting tools complement visualization by offering summarized and detailed reports based on the data collected and analyzed. These reports can be generated automatically on a scheduled basis or on-demand. They provide insights into the overall equipment performance, maintenance activities, and key trends over a specific period. Reports may include information on equipment downtime, failure rates, mean time between failures (MTBF), maintenance costs, and other relevant metrics.
Implementing IoT-Enabled Predictive Maintenance
Let’s look at the steps for implementing IoT-enabled predictive maintenance.
Identifying critical assets and processes
Implementing IoT-enabled predictive maintenance involves identifying critical assets and processes to optimize maintenance activities and minimize downtime. Here are some key steps to identify critical assets and processes in this context:
Asset Inventory
Begin by creating an inventory of all assets and equipment that are relevant to your organization's operations. This includes machinery, vehicles, infrastructure, or any other physical components that are vital to the business.
Historical Data Analysis
Analyze historical maintenance records and performance data of the assets. Look for patterns and trends related to breakdowns, failures, and maintenance requirements. This analysis helps identify assets that frequently require maintenance or have a significant impact on production when they malfunction.
Risk Assessment
Conduct a risk assessment to evaluate the potential consequences of asset failures. Consider the impact on safety, productivity, operational efficiency, customer satisfaction, and regulatory compliance. Assets that pose higher risks or have a significant impact on critical processes should be prioritized.
Sensor and Data Analysis
Determine the type of sensors or monitoring devices required to collect real-time data from the assets. These sensors could measure variables such as temperature, vibration, pressure, or other relevant parameters. Analyze the data collected from the sensors to identify anomalies, patterns, or indicators of potential failures.
Feedback from Maintenance Team
Involve your maintenance team in the identification process. They possess valuable domain expertise and can provide insights based on their experience. The maintenance team can help identify assets that frequently require repairs, cause bottlenecks, or have a history of unexpected failures.
Continuous Improvement
Implement an iterative approach where you continually refine your asset and process identification based on ongoing data collection, analysis, and feedback. As you gather more data and insights, you can optimize your maintenance strategies and adapt to changing conditions.
Selecting and installing appropriate sensors and devices
Implementing IoT-enabled predictive maintenance involves selecting and installing the appropriate sensors and devices to collect and transmit data from various assets and equipment. The selection of sensors and devices depends on the specific maintenance requirements and the assets being monitored. Here are some common sensors and devices used in IoT-enabled predictive maintenance:
- Vibration sensors: Vibration sensors detect abnormal vibrations in machinery, which can indicate potential faults or failures. These sensors measure and transmit vibration data for analysis.
- Temperature sensors: Temperature sensors monitor the operating temperature of equipment and machinery. Deviations from normal temperature ranges can indicate potential issues.
- Pressure sensors: Pressure sensors measure fluid pressure in systems such as hydraulic or pneumatic equipment. Unusual pressure fluctuations can be a sign of problems.
- GPS trackers: GPS trackers provide location information for mobile assets or vehicles. They can be used to track the usage patterns, maintenance history, and predict maintenance needs based on location data.
- Data loggers: Data loggers record and store data over time. They can be used to capture and analyze trends, patterns, or events that may affect maintenance requirements.
During the installation process, it is essential to ensure proper sensor placement and calibration, reliable power supply, and secure data transmission. Integration with a robust IoT platform or a predictive maintenance software solution enables data processing, analytics, and generating actionable insights for maintenance decision-making.
Establishing connectivity and data transmission
Implementing IoT-enabled predictive maintenance requires establishing connectivity and data transmission between various components. Here are the key steps involved in the process:
- Identify sensors and devices: Determine the sensors and IoT devices required to collect relevant data for predictive maintenance. These can include sensors for temperature, pressure, vibration, humidity, or any other relevant parameters based on the equipment or system being monitored.
- Select communication protocols: Choose appropriate communication protocols to facilitate data transmission between the sensors/devices and the central monitoring system. Commonly used protocols include Wi-Fi, Bluetooth, Zigbee, cellular networks (2G/3G/4G/5G), or specialized industrial protocols like MQTT or OPC-UA.
- Establish connectivity: Set up the necessary infrastructure to enable connectivity between the sensors/devices and the central monitoring system. This typically involves deploying gateways, access points, or network infrastructure that supports the chosen communication protocols.
- Data collection and aggregation: Configure the sensors/devices to collect data at regular intervals or in response to specific events. The collected data is then aggregated and packaged for transmission to the central monitoring system. This step may involve preprocessing and filtering the data to reduce noise or extract relevant features.
- Secure data transmission: Implement appropriate security measures to protect the data during transmission. This includes encrypting the data, implementing authentication mechanisms, and ensuring the integrity and confidentiality of the data as it moves through the network.
- Data transmission protocols: Define the protocols for transmitting data from the sensors/devices to the central monitoring system. This could involve establishing a real-time streaming connection or using periodic data uploads based on the available network bandwidth and latency requirements.
- Cloud or edge processing: Decide whether the data should be processed at the edge (near the sensors/devices) or in the cloud. Edge processing involves performing initial data analysis and filtering on the local devices, reducing the amount of data transmitted and minimizing latency.
- Central monitoring system: Develop or deploy a central monitoring system that receives, stores, and analyzes the collected data. This system should have the necessary capabilities to process the incoming data, apply predictive analytics models, and generate maintenance alerts or recommendations based on the analyzed data.
- Continuous improvement: Regularly review the performance of the IoT-enabled predictive maintenance system, analyze the collected data, and refine the algorithms or models used for predictive maintenance. This iterative process helps improve the accuracy of predictions and optimize maintenance schedules.
By following these steps, organizations can establish connectivity and ensure efficient data transmission in an IoT-enabled predictive maintenance system.
Developing and fine-tuning predictive models
Implementing IoT-Enabled Predictive Maintenance involves developing and fine-tuning predictive models that leverage data from IoT devices to proactively identify and prevent potential equipment failures. Here are the key steps involved in this process:
- Define the Problem: Clearly define the maintenance problem you want to address. Identify the equipment or systems you want to monitor and the specific failure modes or performance degradation you want to predict.
- Data Collection: Install appropriate sensors and IoT devices to collect relevant data from the equipment.
- Feature Selection: Analyze the collected data and select the most informative features for predictive modeling.
- Model Selection: Choose an appropriate predictive modeling technique based on the nature of the problem and the available data.
- Model Evaluation: Assess the performance of the trained model using the validation set.
- Model Deployment: Once the model performance is satisfactory, deploy it to a production environment.
- Ongoing Model Maintenance: Continuously monitor the performance of the deployed predictive model.
Remember that successful implementation of IoT-enabled predictive maintenance requires a multidisciplinary approach involving domain expertise, data engineering, machine learning, and continuous collaboration between maintenance teams and data scientists.
Integrating insights into maintenance workflows
Implementing IoT-enabled predictive maintenance involves leveraging data from connected devices to gain insights and optimize maintenance workflows. By integrating these insights into maintenance workflows, organizations can improve equipment reliability, reduce downtime, and minimize maintenance costs.
By integrating insights from IoT-enabled predictive maintenance into maintenance workflows, organizations can transform reactive maintenance into proactive and data-driven approaches. This integration optimizes resource allocation, improves equipment reliability, and ultimately enhances operational efficiency.
Challenges and Considerations in IoT-Based Predictive Maintenance
As we mentioned, IoT-based predictive maintenance have lots of benefits. However, amidst the benefits and opportunities, there are significant challenges and considerations. Let’s now discuss the main challenges.
Data privacy and security
Data privacy refers to the protection of an individual's personal information and ensuring that it is collected, stored, and used in a manner that respects their rights. In the context of IoT-based predictive maintenance, several privacy challenges arise due to the extensive data collection and processing involved. These challenges include:
Data Collection
Predictive maintenance systems rely on vast amounts of data collected from various IoT devices, such as sensors, actuators, and equipment. This data often includes sensitive information about individuals, such as location, usage patterns, and maintenance history. It is crucial to establish clear consent mechanisms and transparent data collection practices to ensure individuals' privacy rights are respected.
Data Storage and Transmission
The massive volume of data generated by IoT devices requires robust storage and transmission mechanisms. Organizations must implement secure data storage practices, including encryption and access controls, to safeguard data against unauthorized access or breaches during transit.
Data Ownership and Control
Determining ownership and control over the data collected through IoT devices can be complex, especially when multiple stakeholders are involved. Clear data ownership policies and agreements must be established to avoid disputes and ensure that individuals' privacy rights are protected.
Third-Party Involvement
IoT-based predictive maintenance often involves partnerships and collaborations with third-party service providers, data analytics companies, and cloud platforms. Sharing data with these entities introduces additional privacy risks. Organizations must carefully assess the privacy practices and security measures of third-party providers to ensure compliance with privacy regulations and protect sensitive data.
User Consent and Transparency
Organizations should obtain explicit user consent for data collection and clearly communicate how the collected data will be used. Users should have control over their data, including the ability to access, modify, and delete their personal information. Transparent privacy policies and practices are essential for building trust with users.
Integration with existing systems and processes
Integration with existing systems and processes is a crucial aspect when implementing IoT-based predictive maintenance solutions. While predictive maintenance offers numerous benefits, such as reduced downtime, improved asset performance, and cost savings, it also presents several challenges and considerations when it comes to integrating with existing systems and processes.
Data compatibility
IoT-based predictive maintenance relies on collecting and analyzing large volumes of data from various sources, including sensors, machines, and other connected devices. One of the primary challenges is ensuring data compatibility between different systems and devices.
Scalability and infrastructure
Integrating IoT-based predictive maintenance solutions into existing systems requires careful consideration of the infrastructure and scalability aspects. Legacy systems may not have the necessary capacity or capabilities to handle the increased data influx from IoT devices.
Legacy system constraints
Existing systems and processes may have limitations or constraints that pose challenges during integration. For example, legacy systems might not provide the necessary interfaces or APIs for direct integration with IoT devices. In such cases, developing custom adapters or middleware to bridge the gap between the legacy systems and IoT infrastructure becomes necessary.
Change management
Integrating IoT-based predictive maintenance solutions often requires changes in processes, workflows, and personnel responsibilities. This change management aspect can be challenging, as it involves training and educating employees, revising standard operating procedures, and ensuring smooth transitions without disrupting ongoing operations.
Ensuring sensor accuracy and reliability
In IoT-based predictive maintenance, ensuring sensor accuracy and reliability is crucial for the overall effectiveness and success of the system. Sensors play a vital role in collecting data from various equipment and assets, enabling predictive analytics and maintenance strategies. However, there are several challenges and considerations involved in ensuring the accuracy and reliability of these sensors. Let's explore some of them:
Sensor Selection
Choosing the right sensors is the first step in ensuring accuracy and reliability. Different types of sensors are available for monitoring different parameters such as temperature, pressure, vibration, humidity, etc. It is essential to select sensors that are suitable for the specific application and can provide accurate measurements consistently.
Calibration and Maintenance
Regular calibration of sensors is necessary to maintain their accuracy. Calibration involves comparing the sensor's measurements with a known reference to identify and correct any deviations. Additionally, routine maintenance procedures should be implemented to keep the sensors in optimal working condition and prevent drift or degradation over time.
Environmental Considerations
The environment in which sensors operate can significantly impact their accuracy and reliability. Factors such as temperature variations, humidity, electromagnetic interference, and physical obstructions can introduce errors or affect sensor performance. Proper shielding, insulation, and environmental monitoring can help mitigate these challenges.
Data Validation and Filtering
Sensor data can be prone to noise, outliers, or intermittent failures. It is crucial to implement data validation and filtering techniques to identify and discard erroneous or inconsistent data points. Statistical methods, signal processing algorithms, and machine learning techniques can be employed to detect and filter out unreliable data, ensuring the accuracy of the overall predictive maintenance system.
Managing the volume and complexity of data
Managing the volume and complexity of data is a critical challenge in IoT-based predictive maintenance systems. With the proliferation of IoT devices and the continuous monitoring of assets, vast amounts of data are generated at an unprecedented rate. This data includes sensor readings, equipment logs, maintenance records, and other relevant information. Effectively handling and processing this data is essential to extract actionable insights and enable proactive maintenance strategies.
The following are key challenges and considerations in managing the volume and complexity of data in IoT-based predictive maintenance:
Data Acquisition and Integration
IoT devices generate massive amounts of data from diverse sources. Ensuring seamless integration and consolidation of this data from various sensors, devices, and systems is a complex task. It requires standardized data formats, protocols, and interfaces to capture and aggregate data efficiently
Data Storage and Management
Storing and managing large volumes of data pose significant challenges. Traditional databases may not be suitable due to their limited scalability and performance. Instead, organizations need to adopt scalable storage solutions like distributed file systems or NoSQL databases.
Data Quality and Preprocessing
Ensuring the quality and reliability of the data is paramount. Data collected from IoT devices may be noisy, incomplete, or subject to anomalies. Preprocessing techniques such as data cleaning, filtering, and outlier detection are necessary to enhance the quality and integrity of the data.
Scalability and Real-time Processing
IoT-based predictive maintenance systems must handle the increasing scale and velocity of data generated in real-time. The architecture should support horizontal scalability, enabling the system to handle a growing number of devices and data sources
Real-World Examples of IoT-Enabled Predictive Maintenance
Let’s look at some examples of how businesses use IoT-enabled predictive maintenance
Case Study 1: Optimizing Fleet Management in a Transportation Company
A logistics company experienced increased maintenance costs and vehicle downtime. These factors impacted business in a negative way, and it was important to find the solution and reduce maintenance costs.
Implementation: the company implemented an IoT-based predictive maintenance system to monitor vehicle health and performance, which helped spot any problems immediately.
Results and Benefits: as a result of IoT-based predictive maintenance system, the company received:
- Reduced maintenance costs and downtime;
- Improved fleet utilization and efficiency;
- Enhanced driver safety and customer satisfaction.
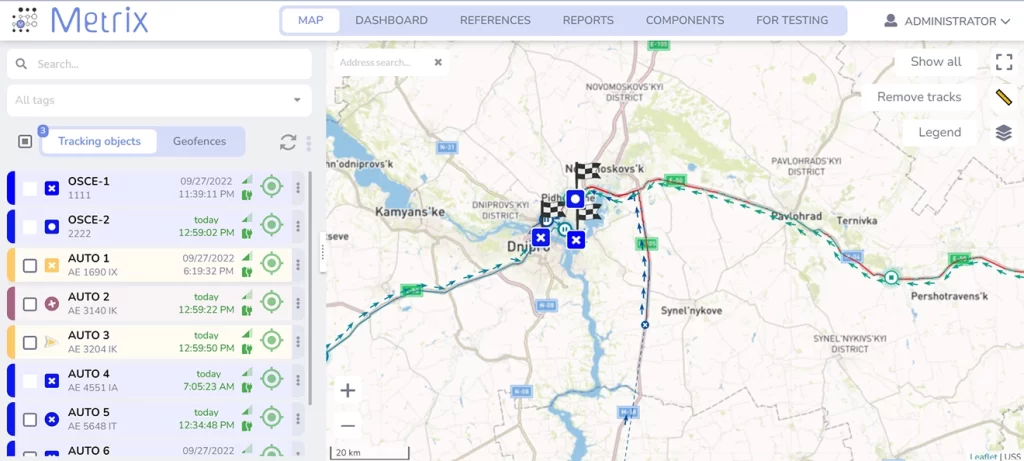
Case Study 2: Enhancing Production Efficiency in a Manufacturing Plant
A manufacturing plant at some point faced frequent equipment breakdowns and, as a result, lost production time. It was necessary to address the problem as soon as possible.
Implementation: the company implemented an IoT-enabled predictive maintenance system to monitor machine conditions and performance.
Results and Benefits: as a result of implementation of IoT-enabled predictive maintenance, the company received the following benefits:
- Increased equipment lifespan and reduced maintenance costs;
- Improved production efficiency and reduced downtime;
- Enhanced decision-making and resource allocation.
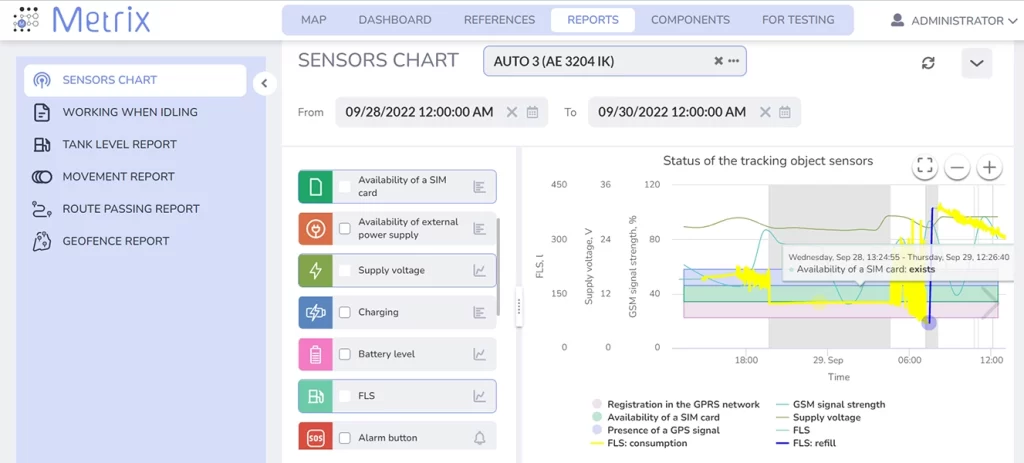
Future Trends in IoT and Predictive Maintenance
What is the future of predictive maintenance IoT? Here are some future trends
Integration of advanced analytics and AI technologies
Predictive maintenance, a key application of advanced analytics in IoT, involves the proactive monitoring of equipment and systems to detect potential failures or malfunctions before they occur.
Artificial Intelligence (AI) technologies, such as machine learning, deep learning, and natural language processing, complement advanced analytics by enabling automated decision-making and the ability to learn from data. AI algorithms can analyze vast volumes of IoT data in real-time, identify patterns and trends, and make predictions or recommendations. This capability is particularly beneficial in predictive maintenance scenarios, where AI algorithms can identify early warning signs of equipment failure, recommend maintenance actions, and optimize maintenance schedules to minimize downtime and maximize asset performance.
The integration of advanced analytics and AI technologies with IoT in predictive maintenance offers several advantages. Firstly, it enables organizations to move from a reactive maintenance approach to a proactive or even predictive one. By continuously monitoring and analyzing IoT data, organizations can anticipate equipment failures, schedule maintenance activities in advance, and avoid costly unplanned downtime.
The rise of digital twins and simulations
The integration of digital twins and simulations into IoT and predictive maintenance has several implications and benefits. Firstly, it enables organizations to gain deep insights into the performance of their assets and predict maintenance requirements accurately. By continuously monitoring the digital twin, companies can detect anomalies, anticipate failures, and plan maintenance activities proactively. This approach helps minimize downtime, optimize resource allocation, and extend the lifespan of assets, leading to significant cost savings.
Secondly, digital twins and simulations enhance the ability to optimize operations and improve overall efficiency. By running virtual scenarios, organizations can identify the optimal settings, configurations, and parameters for their systems. This iterative process enables them to fine-tune operations, reduce energy consumption, optimize supply chains, and streamline production processes. As a result, organizations can achieve higher productivity, reduce waste, and enhance their competitive advantage.
Furthermore, digital twins and simulations facilitate the development and testing of new products and services. Organizations can create virtual prototypes and simulate their performance under different conditions, allowing for rapid iteration and design improvements. This approach accelerates the innovation cycle, reduces development costs, and enhances the quality of final products or services.
However, the widespread adoption of digital twins and simulations also presents challenges. Firstly, it requires significant investments in infrastructure, sensors, connectivity, and data management systems. Organizations need to ensure the availability, reliability, and security of data to maintain accurate digital replicas and perform reliable simulations. Additionally, interoperability and standardization across different systems and platforms are essential for seamless integration and collaboration between stakeholders.
Increased adoption of edge computing and 5G networks
The integration of 5G networks with IoT systems is another transformative trend in the field. 5G offers significantly higher data transfer speeds, lower latency, and increased network capacity compared to its predecessors. These capabilities make it an ideal communication infrastructure for IoT devices, particularly in scenarios that demand ultra-reliable and low-latency connectivity.
By leveraging 5G networks, IoT devices can transmit vast amounts of data in near real-time, enabling faster and more accurate predictive maintenance. For instance, in a smart city environment, 5G-enabled sensors can continuously monitor the condition of critical infrastructure such as bridges, roads, and utility systems. The high-speed connectivity provided by 5G networks ensures that the data from these sensors reaches the analytics platforms rapidly, allowing for proactive maintenance and timely interventions. This not only enhances the safety and reliability of the infrastructure but also minimizes disruptions and improves the overall quality of life for citizens.
Benefits for businesses to explore IoT-based predictive maintenance
Embracing IoT-based predictive maintenance can bring numerous benefits to businesses, including enhanced efficiency and cost savings. Predictive maintenance helps reduce both planned and unplanned maintenance costs. By adopting a proactive approach, businesses can avoid unnecessary scheduled maintenance, thereby saving on labor, spare parts, and associated expenses. By leveraging IoT data for predictive maintenance, businesses can optimize their operational processes. Among other benefits are:
Improved website performance and customer experience
IoT devices integrated into the digital infrastructure can continuously monitor various components of a website, such as servers, databases, networks, and applications. These devices collect real-time data on performance metrics. By analyzing this data using predictive analytics, businesses can identify patterns, detect anomalies, and predict potential failures or bottlenecks.
With this information, organizations can take proactive measures to address issues before they impact website performance. For example, predictive maintenance can alert IT teams about an impending server overload and enable them to allocate additional resources or optimize server configurations in advance.
A fast, reliable, and user-friendly website is crucial for providing an exceptional customer experience. IoT-based predictive maintenance helps businesses achieve this by minimizing service disruptions, reducing response times, and preventing performance issues that can frustrate customers.
Increased sales due to reduced downtime
IoT-based predictive maintenance offers a range of benefits, and reduced downtime stands out as a key factor contributing to increased sales. By leveraging real-time monitoring, accurate analytics, and proactive maintenance strategies, businesses can optimize equipment uptime, allocate resources efficiently, satisfy customers, save costs, and gain a competitive advantage. Embracing this transformative approach not only improves operational efficiency but also drives sales growth and enhances business performance in the long run.
Enhanced ability to focus on core business operations and growth
One of the significant benefits of utilizing IoT-based predictive maintenance is the enhanced ability to focus on core business operations and growth. By leveraging IoT sensors and data analytics, companies can proactively monitor and predict equipment failures or maintenance needs. This proactive approach minimizes unplanned downtime, reduces maintenance costs, and optimizes overall operational efficiency.
Choosing the Right MSP
It might not be easy to choose the right MSP. Here are some things to consider while choosing the right MSP.
Factors to consider when selecting an MSP
When selecting a Managed Service Provider (MSP), it's essential to consider several factors to ensure you choose the right provider that aligns with your business needs. Here are some key factors to consider:
Expertise and Services
Assess the MSP's expertise and range of services. Ensure they have experience in managing the specific technologies, systems, or infrastructure your organization uses.
Scalability and Flexibility
Evaluate the MSP's ability to scale their services according to your business growth and evolving needs.
Service Level Agreements (SLAs)
Review the SLAs offered by the MSP. Assess their commitments regarding uptime, response times, resolution times, and support availability
Security and Compliance
Consider the MSP's approach to security and compliance. Determine if they have robust security measures, such as data encryption, access controls, and threat detection and response protocols.
Cost and Value
Compare the costs and value proposition of different MSPs. Request detailed pricing proposals and consider the value they provide relative to their pricing.
Importance of cultural fit and communication
A strong cultural fit facilitates smoother collaboration, promotes a shared vision, and enhances teamwork. When the MSP understands your organization's culture, it becomes easier for them to adapt to your processes, engage with your employees, and provide services that align with your company's goals. This alignment leads to increased productivity, satisfaction, and overall success.
Effective communication is another vital aspect to consider when choosing an MSP. Communication serves as the foundation for a healthy working relationship. Clear and open communication channels allow for seamless information exchange, enabling both parties to understand expectations, goals, and requirements. Regular and transparent communication ensures that the MSP is updated on any changes in your business, allowing them to respond promptly and provide the necessary support.
Assessing MSPs through references and testimonials
When evaluating MSPs, requesting references allows you to connect with their existing or previous clients. By speaking directly with these clients, you can gain firsthand knowledge about the MSP's capabilities, strengths, and weaknesses. It's essential to ask specific questions related to the services provided, responsiveness, problem resolution, and overall experience working with the MSP. References can offer a realistic perspective on the MSP's reliability, professionalism, and adherence to service level agreements (SLAs).
Testimonials are another valuable source of information when assessing MSPs. These are typically statements or reviews provided by clients who have had positive experiences with the MSP's services. Testimonials often highlight the MSP's ability to meet client expectations, deliver high-quality solutions, and provide excellent customer support. Reading testimonials can give you an overview of the MSP's strengths and the benefits they bring to their clients.
Conclusion
In conclusion, predictive maintenance with the Internet of Things (IoT) has emerged as a powerful solution for optimizing maintenance practices in various industries. By harnessing real-time data from connected devices, predictive maintenance enables organizations to monitor the condition of equipment, identify potential failures before they occur, and schedule maintenance activities proactively.
The integration of IoT devices allows for the collection of vast amounts of sensor data, including temperature, vibration, pressure, and other relevant parameters. Advanced analytics and machine learning algorithms can then analyze this data to identify patterns, anomalies, and potential signs of equipment degradation or failure. By leveraging these insights, maintenance teams can make informed decisions, prioritize resources, and perform necessary repairs or replacements at the right time, reducing downtime, minimizing costs, and optimizing asset performance.
you may also want to read
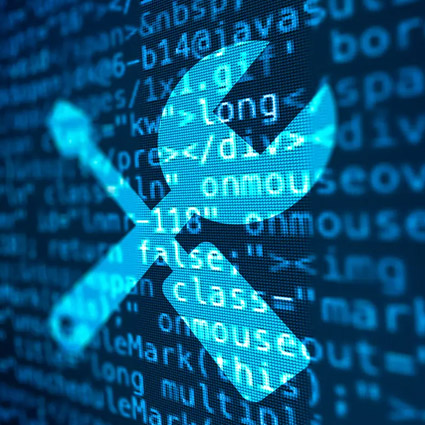
Hire Laravel Framework Developer: Tips and Offers
Do you dream of a dynamic website, a secure eCommerce store, or a new game but lack the resources for...
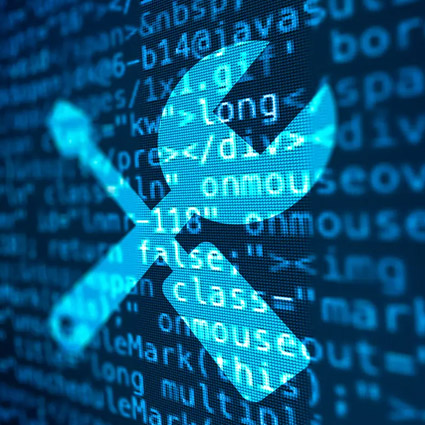
AI in HR: Transforming Human Resources
AI in HR is promised to change the recruitment operational activities dramatically. Despite the changes expected to be grasped by...
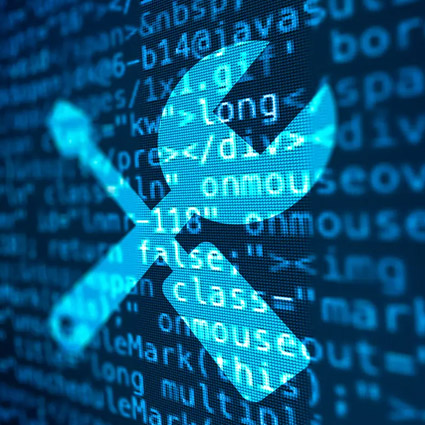
Python Telematic Solutions: Unfolding Capabilities for Fleet Management
The success of transportation and logistics companies is defined by how efficiently their fleet operates. This includes vehicle tracking, route...